DOI: 10.7256/2454-0668.2023.4.44013
EDN: WKMAFQ
Received:
13-09-2023
Published:
16-10-2023
Abstract:
The subject of the research is assessing the risks of performing government contracts. The object of the study is the process of analysis and evaluation of the implementation of government contracts. The study is aimed at developing a methodology that determines the importance and significance of signs that influence the risk of non-fulfillment of government contracts. Research methods were used: data analysis to detect connections and dependencies between various characteristics and the risk of non-fulfillment of government contracts; statistical analysis to obtain an assessment of the impact of each characteristic on the risk of non-fulfillment of contracts and ranking them in order of importance; machine learning to predict the risk of non-fulfillment of government contracts; expert assessments to take into account contextual factors and features, their impact on the importance of features. The main conclusions of the study are the presented methods for assessing the importance of features when analyzing the implementation of government contracts, by using data from various sources, including the register of public procurement of the unified information system (UIS), the register of unscrupulous suppliers (RNP) of the EIS and the SPARK information system. The authors managed to achieve high prediction accuracy (more than 97%) and analyze the most important and significant features. The scientific novelty lies in the fact that the results obtained make it possible to identify and analyze factors from three information systems that influence the risks of non-fulfillment of government contracts. Thus, this study is valuable and important in its field, which contributes to the development of more effective risk management methods and increased efficiency in the implementation of government contracts. The results obtained allow us to identify the factors that have the greatest impact on the risks of non-fulfillment of contracts, which makes the study valuable and important in this area.
Keywords:
government contracts, contract execution, artificial intelligence, machine learning, the importance of signs, classification, risk assessment, feature analysis, the method of support vectors, the SHAP method
This article is automatically translated.
You can find original text of the article here.
The article was prepared as part of the state assignment of the Government of the Russian Federation to the Financial University for 2023 Introduction When analyzing the execution of government contracts, it is important to determine which signs most significantly affect the success of the execution of contracts. The analysis of the signs also allows us to identify factors that may indicate an increased risk of unsuccessful execution of contracts. Knowledge of these factors helps government organizations and stakeholders to take appropriate measures to manage risks, for example, including additional conditions in contracts or the introduction of monitoring and control mechanisms. In addition, the evaluation of features allows you to eliminate unnecessary or insignificant variables that can make noise or distort the results of forecasting. This can improve the quality of the model and increase its accuracy. Thus, the assessment and determination of the importance of features in the analysis of the implementation of government contracts is important from an economic point of view, as it helps to manage risks, optimize costs, improve the quality of forecasts and ensure the explicability of the decision-making process. In the studies of domestic and foreign scientists, various methods of increasing control in the field of procurement are described. The article by M. Y. Aleynikova, D. A. Golovanov examines the issues of various types of internal control of the organization and analyzes the existing model of the control system in the organizations of the budgetary sphere of the Russian Federation. The authors analyzed the risks of violations in the field of procurement and developed models to achieve the strengthening of control procedures and, as a consequence, "timely detection and prevention of violations of contract legislation" [1]. The issues of studying various risks in the field of procurement and contracting are very important for various sectors of the economy. A special place is occupied by features when choosing a supplier, despite the fact that the supplier is determined on a competitive basis. When choosing a supplier, it is important to analyze not only the financial stability of the supplier and his reputation, but also the quality of the services, goods provided by him, and the reliability of supplies. The study of these issues was carried out in the work of M. M. Zolotukhina and N.A. Polovnikova, which "describes the possible consequences of a wrong choice and offers recommendations for the analysis and evaluation of potential suppliers" [2]. The risks of fulfilling contracts are discussed in a wide variety of areas, since purchases are made in all industries. The construction sector covers almost all areas of industry and economy. The construction sector and all activities under contracts "are associated with economic, legal and economic risks affecting the progress and timing of the project, the volume and cost of work, the contract price, the profitability indicators of the contractor", the risk analysis of construction contracts concluded with the municipal customer is considered in the article by Yu. B. Gendlin [3]. The actively developing field of information technology is represented very significantly in the public procurement market. The authors Yu. V. Nemtseva and O. B. Mironets in their study analyzed the development of the market of goods and services offered and requested in the information technology industry. The growth of market volumes requires participants in the procurement process to conduct an in-depth study of the risks arising from the interaction of B2G market participants. Despite the fact that the criteria for selecting contract performers have been approved at the legislative level, the risk management process in the IT industry is nevertheless becoming important. The authors conducted a classification of the types of risks of participants in public procurement in their study, determined methods for assessing the level of risks. The most significant in the article are, significant from the point of view of management, the proposed approaches and methods of risk management [4]. To automate the process of managing the risks of fulfilling contracts is proposed in a study by scientists of the Financial University [5]. The analysis of the reasons for non-fulfillment and termination of contracts, as well as the effectiveness of interaction between the customer and the supplier is considered in the article by A.Ya. Geller [6]. The author points out the dependence between the lack of mechanisms and legislative acts on the part of the customer that would be able to solve the problem of non-fulfillment of the contract and would allow taking into account the effects of environmental factors that do not depend on the participants in the contract process. This would make it possible to fairly distribute the risks of non-fulfillment of the contract between the customer and the contractor. An attempt to take into account the costs and risks of contract violations in the Russian mineral resource complex and the need to apply a mechanism for their insurance is considered in the article by S. M. Lavlinsky, A.A. Panin, A.V. Plyasunov. The authors use the Stackelberg game-theoretic model to obtain the results, which is formulated as a two-level mathematical programming problem. The results of the study are presented in the form of effective solution algorithms based on metaheuristics, which allow in practice to study the properties of the Stackelberg equilibrium on real data, which determine the design of the mechanism for forming economic policy that meets the interests of society. [7] The study of the analysis of the reasons for termination of contracts and the justification of proposals to minimize the risks of termination for purchasers and contract executors using methods of statistical, retrospective analysis, comparative analysis, forecasting elements are considered in the article by D. A. Sozaeva and K.V. Gonchar. Confirmation of the results was obtained on the basis of calculations performed using a system developed in Python. The study confirms the need to study various poorly formalized factors and reasons for termination of contracts. "As a result of the conducted research, the following conclusions were obtained: the probability of termination of state and municipal contracts concluded as a result of public procurement does not directly depend on factors such as savings at auction, competition at auction, the size of the participant's company (small business), as well as the industry within which the purchase is carried out." [8]
A special place in the procurement system is occupied by the state defense order, which is carried out in accordance with the Federal Law "On the State Defense Order" dated 29.12.2012 No. 275-FZ. The article by E. V. Chernyaev and V. A. Khaitbaev considers "various approaches to the assessment of risk factors in the system of program-target planning of the state defense order, and also substantiates the need to use probabilistic methods for assessing the risks of fulfilling contracts of the state defense order on the basis of cooperation built using hierarchical models" [9]. It should be noted that the analysis of the risks of fulfilling government contracts is relevant and widely sanctified in the research of foreign scientists. Thus, the article [10] explores the influence of various factors on the success of contractors in providing tenders for construction projects in the North-Central part of Nigeria. The authors of the article conducted a study to find out which factors can positively or negatively affect the results of tenders in the construction industry of this region. To do this, an analytical approach was used, based on questionnaires and interviews with representatives of contractors who already have experience in providing tenders. In the course of the study, the authors identified several factors that influence the success of contractors in providing tenders. One of these factors is the experience of providing tenders, since contractors with more experience can be more successful in attracting customers and providing proposals. They also found that the reputation of the contractor is of great importance, as clients tend to choose contractors with a good reputation and successful past experience in project execution. Another factor identified in the article is the financial stability of contractors. The authors point out that contractors with strong financial indicators have more opportunities to participate in competitions and win tenders. Lu S. and Wang H. present a study that reveals how political connections exploit shortcomings in public procurement institutions in China [11]. The article emphasizes that political ties can facilitate access to information about public tenders and provide advantages in obtaining contracts. For example, contractors with political connections may have access to information about upcoming tenders and assistance in drawing up competitive offers. Such connections can also help to exclude competitors from the tender review process by using influence on the decisions of the personnel responsible for issuing contracts. This study may be important for improving the public procurement system and increasing transparency and fairness in this area. The research presented in the article [12] allows us to consider the analysis of challenges in the field of placing orders for construction projects in Iraq. The authors also consider the factors affecting the efficiency of the order placement process and offer some recommendations for improving this process. One of the main challenges identified in the article is related to corruption and lack of transparency in the system of placing orders for construction projects in Iraq. The authors point out that many companies face problems related to bribery and lobbying when trying to obtain government contracts. This not only creates unequal conditions for various participants, but also leads to misuse of public funds and poor quality of construction projects. Duguay R., Rauter T., and Samuels D. investigates the impact of open data on public procurement [13]. The authors are based on the conducted research to assess how open data affects various aspects of public procurement. They consider the advantages and challenges associated with the use of open data in the public procurement process, as well as their impact on efficiency, transparency and competition in this area. The article emphasizes that open data can improve the availability of public procurement information and make it more understandable and accessible to all stakeholders. This study can be a valuable resource for policy makers and practitioners involved in public procurement in evaluating and implementing open data in this area. Nani D. A. and Ali S. investigate various aspects, such as the organization of the procurement process, technological support, legal environment and work culture [14]. They conducted an empirical study among various Indonesian local governments to determine the factors affecting the effectiveness of e-procurement systems. As a result of the study, the authors identified several key factors that significantly affect the effectiveness of electronic procurement systems in Indonesian local governments. One of the main factors is the organizational structure and internal processes, including joint procurement management and cooperation between various stakeholders. Technological support has also become a factor affecting the effectiveness of e-procurement systems. The authors found that modern technologies, such as cloud computing and mobile applications, significantly increase the efficiency of the procurement process. The legal environment also plays an important role in the effectiveness of e-procurement systems. Work culture and acceptance of changes are also essential for the successful implementation of electronic procurement systems. The authors found that organizations with an open and innovative work culture are more likely to successfully implement and use electronic procurement systems. The analysis of the above-described studies confirms the relevance of the study of risk assessment of government contracts. However, it should be noted that each of the authors examines certain signs that affect the performance of government contracts. Of these , the following main risks can be identified: 1. Financial risks that can be assessed as the financial performance of the counterparty, the presence of debts to suppliers and employees, the ability to fulfill financial obligations. 2. Experience and qualifications of the counterparty. It is important to analyze the counterparty's experience in implementing such projects, the availability of the necessary qualifications and certifications, as well as its reputation in the market. 3. Technical risks. It is important to assess the state of the counterparty's technical base, the availability of the necessary equipment, technological processes and qualified employees. 4. Legal risks, where it is necessary to study possible legal problems related to the contract, the specifics of legislation, the possibility of disputes and their consequences.
That is, the risk assessment of the execution of government contracts should be comprehensive and take into account all of the above factors in order to reduce the likelihood of problems and ensure the successful implementation of the project. At the same time, it is very important to understand which feature can have a stronger impact on the performance of the contract in a particular area. Therefore, a methodology is needed to assess the importance of features in the analysis of the implementation of government contracts. This is the main novelty of this study. Collecting data for research It should be noted that the analysis of scientific literature made it possible to identify the most important aspects in assessing the implementation of government contracts. These include: the financial performance of the counterparty; his experience and qualifications; technical base and legal reputation. Based on this, a set of data from various sources was collected for further analysis: 1. The Register of Public Procurement of the Unified Information System (UIS) (https://zakupki.gov.ru /). 2. Register of Unscrupulous Suppliers (RNP) EIS (https://zakupki.gov.ru/epz/dishonestsupplier/search/results.html ). 3. SPARK Information System (https://spark-interfax.ru /). Each of these sources, RNP, SPARK and EIS, provided a table containing deeply detailed information on each individual element of the contract. These data have been carefully collected and combined into a single table for ease of analysis and processing. So the following signs were obtained from the EIS: • Number of the contract registry entry • Customer: name • Customer: INN • Customer: Checkpoint • Budget level • Source of financing of the contract: name of the budget • Source of contract financing: name/type of extra-budgetary funds • Order placement method • Number of the auction notice • Date of summing up the results of determining the supplier (contractor, contractor) • Details of the document confirming the basis of the conclusion of the contract • Contract: Date Contract: Number Subject of the contract • Contract price • Budget classification code • KOSGU • KVR • Purchase Identification Code (ICD) • Object of purchase: name of goods, works, services • Purchase object: item code • Object of purchase: unit price, rubles • Object of purchase: quantity of goods delivered, works performed, services rendered • Object of purchase: amount, rubles • Information about suppliers (performers, contractors) under the contract: name of the legal entity (full name of the individual) • Information about suppliers (performers, contractors) under the contract: TIN • Information about suppliers (performers, contractors) under the contract: KPP • Date the record was last modified • Date of execution of the contract: under the contract • Status
• Reason for termination The data from the EIS were consistently enriched with additional features from two sources: - Register of unscrupulous suppliers; - SPARK Interfax products. Enrichment was carried out in both cases by the key field – the INN of the supplier. The specified TIN was used in the SPARK service as an argument for uploading data. Initially, a list of unique INN was uploaded to SPARK, on the basis of which a sample was created. A certain number of INN (several hundred) it was not found in the SPARK database, so contracts with the specified INN of suppliers were later removed from the analysis. Then, based on the samples uploaded to SPARK, custom analytical reports for all supplier companies were generated and uploaded. A total of 124 signs were uploaded. We will not list everything, we will note only a number that reflect the financial performance of the counterparty. In this case it is: · name; · registration number; · head – INN; · age of the company, years; · co-owners, priority; · previously used TIN; · credit limit, RUB; · the amount of pending claims in the role of the defendant, RUB; · amount of enforcement proceedings; · intangible assets, RUB; · fixed assets , RUB; · profitable investments in tangible assets, RUB; · long-term financial investments, RUB; · deferred tax assets, RUB; · other non-current assets, RUB; · non-current assets, RUB; · stocks, RUB; · net assets, RUB;
· VAT on purchased valuables , RUB; · accounts receivable, RUB; · short-term financial investments, RUB; · long-term liabilities, RUB; · borrowed funds (short-term), RUB; · accounts payable, RUB; · own working capital, RUB; · equity, RUB; · total debt, RUB; · revenue, RUB; · gross profit (loss), RUB; · net profit (loss), RUB; · profit margin before tax and interest - (EBITM), %; · Return on assets (ROA), %; · Return on equity (ROE), %; · quick liquidity ratio, %; · absolute liquidity ratio, % · and others. The data set also included information from the Register of Unscrupulous suppliers. As a result of the work of the data parser , it was possible to collect the following data: • TIN (similar to TIN) of an unscrupulous supplier in the RNP • The number of entries in the RNP by supplier for all time • Date of the supplier's first inclusion in the RNP • Date of the last exclusion of the supplier from the RNP • Supplier status • Type of supplier • Date of last inclusion in the RNP After uploading the data, a number of derived features were developed that can better assess the dynamics of the behavior of a particular supplier:
• Number of entries in the RNP in 2018 • Number of entries in the RNP in 2019 • Number of entries in the RNP in 2020 • Number of entries in the RNP in 2021 • Number of entries in the RNP in 2022 • The number of entries in the RNP in 2023 • Number of entries based on: Termination of the contract • Number of entries based on: Termination of the contract in case of unilateral refusal of the supplier • Number of entries based on: Termination of the contract by court decision • The number of entries on the basis of: Avoidance of the sole participant from the conclusion of the contract • The number of entries on the basis of: The winner's evasion from the conclusion of the contract • Number of entries based on: Evasion of the participant after the winner from the conclusion of the contract • Approximate date of exclusion from the RNP In the process of data processing, an extensive table with a size of 83834 by 184 was created. Assessment of the importance of signs The resulting data set is used as the basis for constructing a model for solving the binary classification problem: assessing the risk of non-fulfillment of the contract on time. As a result of applying machine learning methods for this task, the following results were obtained (Table 1). Table 1. Algorithms and metrics of the task of assessing non-fulfillment of the contract Model | Accuracy | Risk probabilities | Logistic regression | 97,01% | Either have very high values or very low | Decision Tree | 97,89% | Only unambiguous "yes" and "no" | The method of support vectors |
97,88% | Distributed over the entire interval from 0 to 1. | The support vector machine (SVM) method was used as the basis for modeling the problem of assessing non-fulfillment of the contract. The accuracy metric on the test data reaches a value of 97.88%, which is slightly less than that of the decision tree, but this approach allows you to assess risks more flexibly. The probability distribution of risks for SVM is shown in Figure 1. 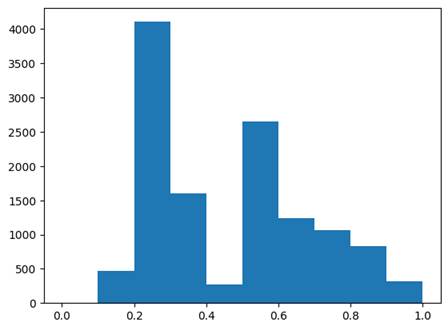 Figure 1. Probability distribution of contract risks by the support vector method The analysis of the presented histogram shows that the probabilities of risks under contracts are not bimodal along the boundaries and, in general, are unevenly distributed. At the same time, a high proportion of contracts are classified as "Contract terminated" with a probability close to 0.5. It is worth noting that these results are not the only criteria for evaluating the quality of the model. An important component in evaluating the effectiveness of the model is its interpretability, that is, the ability to understand on the basis of which data and how the model makes its decisions. This is especially true when working with large amounts of data, when it is necessary to determine which features have the greatest impact on the decision-making model. The SHAP method was used to analyze the contribution of each of the features to decision-making by the model [15]. This method is based on the principles of game theory and allows the analysis of the individual contribution of each feature using statistical methods. This approach allows not only to evaluate the effectiveness of the model, but also to understand which parameters have the greatest impact on its decisions. This is important for further improving the model and clarifying its operation, as well as for a deeper understanding of the processes that occur in the data. Figure 2 shows a graphical analysis of the influence of twenty key characteristics on the final solution of the model. Each of the signs is colored in a certain color depending on its size. The blue color indicates the minimum value of the attribute, and the red color indicates the maximum. The horizontal axis shows the contribution of each attribute to the average result. 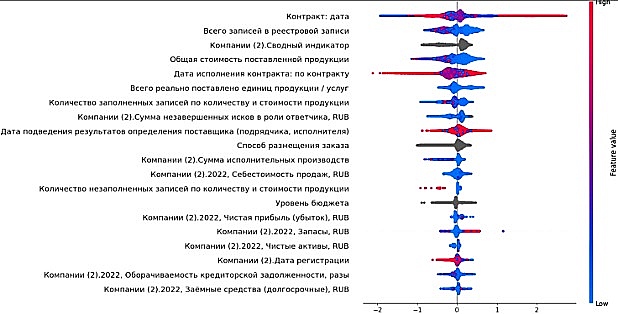 Figure 2. Graphical analysis of the impact of twenty key characteristics Some characteristics, such as borrowed funds, debt turnover, cost of sales and the date of summing up the results, are distributed almost evenly and painted in the same color. This indicates that these characteristics do not have a significant impact on the outcome of the purchase. They are present in both successfully completed and interrupted contracts, and the uniformity of their color markings indicates that they do not play a decisive role in determining the outcome. However, other signs, such as the registration date, inventory, and the number of completed records, are displayed in different colors on the chart. This suggests that these characteristics have a significant impact on the forecast of the model. For example, an increase in stocks leads to a positive shift in the forecast, and their decrease leads to a negative one. In the case of the registration date and the number of completed records, an inverse relationship is observed. So, the earlier the company was registered and the more records it filled out, the higher the probability of successful completion of the contract. This may be due to the fact that older and more active companies usually have more experience and resources to fulfill contracts. The asymmetric distribution of most of the other signs indicates that they make a significant contribution to predicting the outcome. For example, the sign "Total units provided" most often lowered expectations, while the signs "Amount of pending claims" and "Summary indicator" on the contrary increased them. The gray color of these features on the diagram indicates that they are categorical and cannot be converted into quantitative values. The interpretation of such parameters is based on the form of their distribution. The parameters "Contract Execution Date" and "Contract Date" are important elements for forecasting the model. They have a non-uniform shape and color, which indicates the absence of a linear relationship between the parameter value and its contribution to the forecast. Instead of having a direct correlation, these parameters function by means of a piecewise function that gives different results at different intervals. In Figure 3, you can see a clear division into periods, each of which has its own interpretation. Horizontal lines indicate that many contracts have chosen the same period as the completion date. This may be due to certain seasonal factors or standard deadlines for the execution of contracts. Diagonal lines indicate that contracts are planned to be executed after a fixed period of time after the conclusion. This may be due to certain requirements for the execution of contracts or the terms of delivery of goods or services.
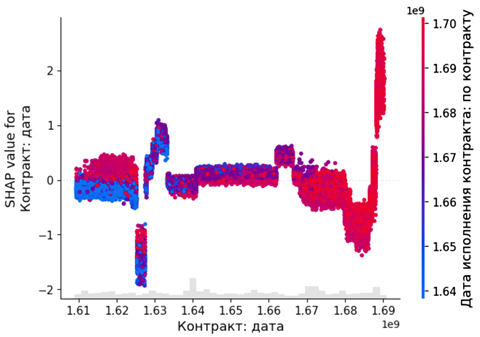 Figure 3. Probability of execution depending on the date of conclusion of the contract with differentiation by date of execution Figure 4 shows a positive outcome, where most factors point in the positive direction. However, the number of entries and the total cost have a negative impact. This allows us to conclude that the large volume and high cost of the purchase reduce the chances of its successful completion. 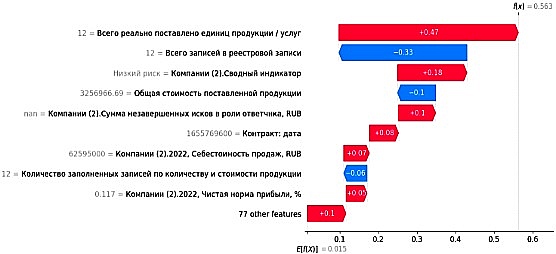 Figure 4. Analysis of special cases of model prediction On the other hand, Figure 5 presents an example with a negative decision, where most factors reduce the probability of a successful outcome. Only the low risk of the company and the high volume of revenue and assets have a positive effect on the outcome, but their contribution is not proportional to the influence of other factors, such as the number of blank entries in the public procurement. This highlights the complexity of predicting outcomes based on multiple factors and the importance of taking into account all significant parameters. 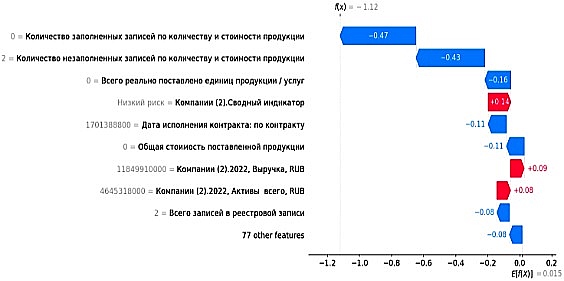 Figure 5. Analysis of special cases of model prediction Based on the analysis , the following conclusions can be formulated: 1. A number of characteristics, including borrowed funds, debt turnover, cost of sales and the date of summing up the results, do not have a significant impact on the final decision of the model, without playing a key role in determining the outcome of the purchase. 2. While some signs, such as the date of registration, stocks and the number of completed records, significantly affect the predicted outcome. Their changes can lead to a positive or negative shift in the forecast. 3. The indicators "Total number of units provided", "Amount of pending claims" and "Summary indicator" make a significant contribution to predicting the outcome. They are categorical features and cannot be converted into quantitative values. 4. Parameters such as "Contract Execution Date" and "Contract Date" play an important role in predicting the outcome, functioning through a piecewise function that gives different results at different intervals. 5. The time of contract execution may be related to seasonal factors or standard deadlines for the execution of contracts, as well as certain requirements for the execution of contracts or terms of delivery of goods or services. 6. Large volume and high purchase price may reduce the probability of successful execution of the contract. 7. Predicting outcomes based on multiple factors is a complex task that requires taking into account all significant parameters. Conclusion As a result, the created machine learning model demonstrates efficiency in predicting the outcomes of public procurement using a variety of parameters. Parameters such as the registration date, inventory, and the number of completed records are important. Categorical features, as well as the terms of execution of the contract and the date of its conclusion are important for forecasting. It should be noted that a large volume and high purchase price may reduce the probability of successful execution of the contract. Despite the complexity of the task of predicting the outcomes of public procurement, which requires taking into account many factors, the developed machine learning model shows good results. The use of such a model can become a tool for optimizing the public procurement process, increasing its transparency and efficiency, as well as for preventing potential risks and problems.
References
1. Aleynikova, M. Yu. & Golovanov, D. A. (2022). Models for improving the system of internal control of public procurement in the Russian Federation. Managerial accounting. 7, 12-19. doi:10.25806/uu7202212-19
2. Zolotukhina, M. M., & Polovnikova, N.A. (2023). Risks in choosing suppliers and concluding contracts. Economics and Business: Theory and practice, 8(102), 86-90.
3. Gendlina, Yu. B. et al. (2022). Risks of construction contracts concluded with a municipal customer. Management and economics of the national economy of Russia, 113-120.
4. Nemtseva, Yu. V., & Mironets, O. B. (2022). Risk management of information technology companies in the B2G market. Managerial accounting, 12, 100-109.
5. Korchagin, S.A., et al. (2023) Decision support system for issuing bank guarantees based on contract execution forecasting using machine learning methods and parsing technologies. Modern science-intensive technologies, 7, 41-47.
6. Geller, A. Ya. (2022). Analysis of the reasons for termination of contracts in the system of state and municipal procurement of the Russian Federation. Bulletin of the University, 5, 5-12.
7. Lavlinsky, S. M., Panin, A. A., & Plyasunov, A.V. (2022). The model of economic policy formation taking into account transaction costs and insurance of contract break risks. Discrete analysis and operations research, 29(3), 45-63. doi:10.33048/Dayo.2022.29.738
8. Sozaeva, D. A., & Gonchar, K. V. (2022). Investigation of the risks of termination of contracts concluded based on the results of public procurement. Problems of risk analysis, 19(3), 74-85.
9. Chernyaev, E. V., & Khaytbaev, V. A. (2022). Probabilistic methods of risk assessment in the system of program-target planning of the state defense order using hierarchical models. Applied Economic research, 3, 24-29.
10. Muhammed, A. O., Muhammed, A. A., Yakubu, H. A., Suleiman, A., & Adam, A. (2022). Assessment of factors affecting contractors tendering success for construction projects in North-Central Nigeria. International Journal of Real Estate Studies, 16(1), 87-99. Retrieved from https://doi.org/10.11113/intrest.v16n1.155
11. Lu, S., & Wang, H. (2022). How political connections exploit loopholes in procurement institutions for government contracts: Evidence from China. Governance. doi:10.1080/14719037.2013.770056
12. Hamza, S. A., Rasheed, S., & Hussein, A. (2022). Procurement challenges analysis of Iraqi construction projects. Journal of the Mechanical Behavior of Materials, 31(1), 112-117. doi:10.1515/jmbm-2022-0012
13. Duguay, R., Rauter, T., & Samuels, D. (2023). The impact of open data on public procurement. Journal of Accounting Research, 61(4), 1159-1224.
14. Nani, D. A., & Ali, S. (2020). Determinants of Effective E-Procurement System: Empirical Evidence from Indonesian Local Governments. Jurnal Dinamika Akuntansi Dan Bisnis, 7(1), 33-50. doi:10.24815/jdab.v7i1.15671
15. Lundberg S. M. & Lee S. I. (2017). A unified approach to the interpretation of model forecasts. Advances in Neural Information processing Systems, 30. doi:10.48550/arXiv.1705.07874
First Peer Review
Peer reviewers' evaluations remain confidential and are not disclosed to the public. Only external reviews, authorized for publication by the article's author(s), are made public. Typically, these final reviews are conducted after the manuscript's revision. Adhering to our double-blind review policy, the reviewer's identity is kept confidential.
The list of publisher reviewers can be found here.
The subject of the study. Based on the title, the article should be devoted to the methodology for assessing the importance of features in analyzing the implementation of government contracts. The content of the article, in general, corresponds to the stated title. The research methodology is based on the use of classical scientific methods (analysis and synthesis) and machine learning methods. The author claims that in the process of data processing, an extensive table with a size of 83834 by 192 was created. However, even its structure is not presented in the text. It is unclear exactly what was received by the author? The relevance of the study of issues related to the implementation of government contracts is beyond doubt, as it has both tactical and strategic importance, including in the context of ensuring the achievement of the national development goals of the Russian Federation set by the President of Russia until 2030. At the same time, it should be noted that the request of the potential readership exists for specific practice-oriented studies containing justification of the identified problems and recommendations for their solution. Scientific novelty is partially present in the materials submitted for review. In particular, the results of the evaluation of the probability of execution depending on the date of conclusion of the contract with differentiation by the date of execution. It seems that the extensive table developed by the author with a size of 83834 by 192 has scientific novelty, but neither its structure nor its meaning is clear from the text. This should be clarified so that the potential reader does not have any questions. Style, structure, content. The style of presentation is scientific. The structure of the article was not built by the author, which became a key factor in the chaotic presentation of the results of the author's research without consistent structural and logical connections. When finalizing the article, it is necessary to eliminate this problem. Also, taking into account the request of the potential readership for specific practical research, it is necessary to justify the existing problems of fulfilling government contracts and ways to solve them. Bibliography. The author has compiled a bibliographic list of 10 sources, including both domestic and one foreign scientific publication. The author is recommended to increase the review of sources, especially those published abroad. This will significantly enhance the quality of the methodological base, as well as create prerequisites for substantiating the scientific novelty of the text. Moreover, it will be possible to form any practical recommendations based on foreign experience, the analysis of which is not presented in the current version of the article. Appeal to opponents. Despite the bibliographic list that has been formed, there is no scientific discussion on them. The elimination of this remark will also make a positive contribution to the formation of scientific novelty. Conclusions, the interest of the readership. Taking into account all the above, the article requires revision, after which the issue of the expediency of publication can be resolved. In its current form, the article may be of interest to the readership, but taking into account comments will make it possible to multiply it and increase the circle of interested persons.
Second Peer Review
Peer reviewers' evaluations remain confidential and are not disclosed to the public. Only external reviews, authorized for publication by the article's author(s), are made public. Typically, these final reviews are conducted after the manuscript's revision. Adhering to our double-blind review policy, the reviewer's identity is kept confidential.
The list of publisher reviewers can be found here.
The reviewed article is devoted to the study of signs affecting the performance of government contracts, identifying the most important of them, which have a significant impact on the results of forecasting the success of the execution of state contracts. The research methodology is based on the application of mathematical modeling and machine learning methods, the construction of a model for assessing the risk of non-fulfillment of government contracts on time using logistic regression methods, decision trees and support vectors. The authors rightly attribute the relevance of the work to the fact that assessing and determining the importance of signs in analyzing the implementation of government contracts is important from an economic point of view, since it helps to manage risks, optimize costs, improve the quality of forecasts and ensure the explainability of the decision-making process, which undoubtedly affects national security. The scientific novelty of the work, according to the reviewer, consists in the machine learning model developed by the authors, which demonstrates the effectiveness of predicting public procurement outcomes using a variety of parameters. Structurally, the following sections are highlighted in the article: Introduction, Data collection for research, Assessment of the importance of features, Conclusion, Bibliography. The advantages of the publication include a detailed description of the initial data for the study, an accessible presentation of the essence of the study, the techniques used to convert the initial information into the results obtained, as well as their visualization. The text of the article is illustrated with the table "Algorithms and metrics for evaluating non-fulfillment of a contract" and several figures: "Probability distribution of contract risks using the support vector method", "Graphical analysis of the influence of twenty key characteristics", "Probability of execution depending on the date of conclusion of the contract with differentiation by date of execution", "Analysis of special cases of model prediction". Based on the results of the study, specific conclusions were formulated, including seven points, and the Conclusion reflects the classification of signs according to their importance for analyzing and predicting the success of government contracts. The authors position the practical significance of the obtained machine learning model as a developed tool for optimizing the public procurement process, increasing its transparency and efficiency, as well as for preventing potential risks and problems. The bibliographic list includes 15 sources – scientific publications on the topic in Russian and English. The text of the publication contains targeted references to the list of references confirming the existence of an appeal to opponents. Of the reserves for improving the article, the following should be noted. Firstly, the publication does not specify which software tools or machine learning platforms were used to process the data. Secondly, Figures 4 and 5 have the same names, and clarification of their names is required. The topic of the article is relevant, the material reflects the results of the research conducted by the authors, contains elements of increment of scientific knowledge, corresponds to the topic of the journal "National Security / nota bene", may arouse interest among readers and is recommended for publication taking into account the expressed wishes.
|